go-wcop.org รีวิวบริการกู้เงินออนไลน์ผ่านแอพกู้เงินหรือสินเชื่อบุคคล ทั้งยังมีการกู้เงินนอกระบบด้วยและมาพร้อมบัตรกดเงินสดเช่นกัน
สนใจกู้เงินด่วนออนไลน์ง่ายๆ ผ่านสินเชื่อบุคคล ผ่านการทำบัตรกดเงินสด หรือโหลดแอพกู้เงินเพื่อยืมเงินในแอพธนาคารปี 67
กู้เงินออนไลน์
รีวิวทางสมัครกู้เงินออนไลน์ผ่านสินเชื่อต่างๆ ผ่านแอพมากมายหรือไปสมัครกู้ยืมเงินนอกระบบ และยังมีบัตรกดเงินสดให้เลือกอีกด้วย
วิธีการตรวจสอบแอพกู้เงินออนไลน์ผ่านเว็บไซต์ธนาคารแห่งประเทศไทย
การยืมเงินในอนาคตเรียกได้ว่าเป็นสิ่งที่ทุกคนต่างไม่ต้องการเพราะทุกคนไม่ต้องการที่จะเป็นหนี้ แต่เราก็ไม่สามารถหลีกเลี่ยงได้เพราะเมื่อเราต้องการใช้เงินด่วน เรามักจะมองหาวิธีกู้เงินที่มาจากแอพกู้เงินออนไลน์หรือการกู้เงินผ่านสถาบันการเงินเพราะการกู้เงินแบบนี้เป็นการกู้เงินที่ถูกกฎหมายแต่ก็จะมีบางแอพกู้เงินออนไลน์ที่เป็นแอพผิดกฎหมายมีการคิดอัตราดอกเบี้ยเกินจริงและมีวิธีการทวงหนี้โหด คุณควรจะตรวจสอบแอพกู้เงินออนไลน์ให้ดีเสียก่อนซึ่งวิธีการตรวจสอบแอพกู้เงินออนไลน์ว่าเป็นแอพถูกกฎหมายไหม อันดับแรกเลยคุณจะต้องตรวจสอบว่า กู้เงินออนไลน์ได้จริงมีใบอนุญาตของกระทรวงการคลังไหม สาเหตุที่คุณจะต้องตรวจสอบเพราะเมื่อคุณได้มีการกู้เงินด่วนออนไลน์ผ่านแอพจะต้องทำการกรอกชื่อ นามสกุล เลขที่บัตรประจำตัวประชาชน 13 หลักลงไปในแอพกู้เงินออนไลน์ หากแอพกู้เงินออนไลน์เป็นแอพยืมเงินนอกระบบด่วนของมิจฉาชีพคุณอาจจะโดนขโมยข้อมูลส่วนตัวไปใช้ได้ เพื่อความปลอดภัยคุณควรจะตรวจสอบก่อนว่า แอพเงินกู้ออนไลน์อยู่ภายในการกำกับของธนาคารแห่งประเทศไทยไหมซึ่งวิธีตรวจสอบก็สามารถตรวจสอบชื่อกู้เงินออนไลน์ได้ที่เว็บไซต์ของธนาคารแห่งประเทศไทย เมื่อคุณเข้าไปที่เว็บไซต์แล้วก็สามารถใส่ชื่อเพื่อทำการตรวจสอบแอพกู้เงินออนไลน์ที่คุณต้องการทราบได้เลย ถ้ามีแอพกู้เงินออนไลน์นั้นโชว์ที่หน้าเว็บไซต์ก็แสดงว่า เป็นแอพกู้เงินออนไลน์ที่ถูกกฎหมายได้รับอนุญาตจากธนาคารแห่งประเทศไทยเรียบร้อยแต่ถ้าแอพกู้เงินด่วนออนไลน์ไม่แสดงชื่อนั่นหมายความว่า แอพกู้เงินออนไลน์เป็นแอพที่ผิดกฎหมายให้หลีกเลี่ยงในการกู้เงินด่วนออนไลน์
อัตราดอกเบี้ยแอพกู้เงินออนไลน์ที่ถูกกฎหมายมีการกำหนดไว้เท่าไร
เมื่อคุณทำการขอสินเชื่อออนไลน์ควรที่จะต้องทราบก่อนว่า สินเชื่อแต่ละตัวมีการกำหนดอัตราดอกเบี้ยไม่เกินร้อยละเท่าไรเพราะถ้าแอพกู้เงินออนไลน์มีการกำหนดอัตราดอกเบี้ยเกินกว่าที่ธนาคารแห่งประเทศไทยได้กำหนดไว้ จะเป็นแอพกู้เงินออนไลน์ที่ผิดกฎหมายในทันที สำหรับแอพกู้เงินออนไลน์ที่ถูกกฎหมายจะต้องปฏิบัติตามข้อบังคับของธนาคารแห่งประเทศไทยที่ว่า อัตราดอกเบี้ยจะต้องไม่เกินตามที่กฎหมายได้กำหนดไว้โดยสินเชื่อส่วนบุคคลจะต้องมีอัตราดอกเบี้ยไม่เกิน 25 ต่อปีแต่ถ้าเป็นสินเชื่อรถจะต้องไม่เกินร้อยละ 24 ต่อปีซึ่งสินเชื่อรถเป็นสินเชื่อที่มีหลักประกันซึ่งจะใช้รถในการค้ำประกันโดยวิธีคิดดอกเบี้ยเงินกู้จะแตกต่างจากสินเชื่อส่วนบุคคล, การขอสินเชื่อออนไลน์อย่างสินเชื่อ Nano Finance หรือสินเชื่อรายย่อยเพื่อการประกอบอาชีพเป็นสินเชื่อรายย่อยที่ใช้ในการประกอบอาชีพจะต้องมีอัตราดอกเบี้ยไม่เกินร้อยละ 33 ต่อปีโดยวิธีคิดดอกเบี้ยเงินกู้จะคิดแบบลดต้นลดดอกเมื่อคุณชำระหนี้ไปเรื่อยๆ ดอกเบี้ยจะถูกลงซึ่งถ้าเดือนไหนที่ต้องการนำเงินมาโปะก็จะส่งผลให้คุณหมดหนี้ได้เร็วขึ้นซึ่งจะแตกต่างจากการที่อัตราดอกเบี้ยคงที่ส่งผลให้คุณจะต้องจ่ายดอกเบี้ยเท่ากันทุกเดือนซึ่งส่นใหญ่แล้วจะเป็นสินเชื่อเช่าซื้อรถยนต์โดยคุณจะต้องนำเงินมาจ่ายในทุกเดือนซึ่งจะมีการชำระเงินต้นพร้อมดอกเบี้ยจนหมดอายุสัญญาของสินเชื่อนั้น
แอพกู้เงินออนไลน์ส่วนใหญ่จะไม่ใช้หลักทรัพย์ในการค้ำประกันจริงไหม?
การกู้เงินออนไลน์ส่วนใหญ่จะไม่ใช้บุคคลหรือหลักทรัพย์ในการค้ำประกัน ทำให้คุณไม่จำเป็นต้องไปขอช่วยให้บุคคลอื่นทำการค้ำประกันให้กับคุณซึ่งการไปให้ผู้อื่นทำการค้ำประกันให้อาจจะสร้างความลำบากใจให้กับบุคคลนั้น ส่วนหลักทรัพย์ที่ได้มีการค้ำประกันนั้นก็สามารถจะเก็บไว้เป็นเงินทุนสำรองเมื่อเราต้องการเงินด่วนเพราะหลักทรัพย์ที่คุณใช้ในการขอกู้เงินด่วนออนไลน์มักจะมีมูลค่าสูง ถ้าใช้ในการค้ำประกันจะส่งผลในคุณได้วงเงินสูงขึ้น ส่วนใหญ่แล้วแอพกู้เงินออนไลน์จะใช้ในการสลิปเงินเดือนของผู้สมัครเงินด่วนออนไลน์พร้อมสเตทเม้นท์ย้อนหลัง 6 เดือนมากกว่าการใช้บุคคลหรือหลักทรัพย์ในการค้ำประกัน แต่ก็จะมีสินเชื่อบางประเภทที่ใช้หลักทรัพย์ในการค้ำประกันอย่างสินเชื่อบัตรกดเงินสดบางตัว-สินเชื่อบ้านเพื่อให้สถาบันการเงินมีความมั่นใจว่า คุณสามารถชำระหนี้ได้ตรงเวลาตามที่กำหนด หากคุณไม่มีการชำระหนี้ทางสถาบันการเงินก็อาจจะยึดหลักทรัพย์ของคุณเพื่อนำการขายทอดตลาด การใช้แอพกู้เงินออนไลน์ส่วนใหญ่แล้วจะเป็นการขอสินเชื่อออนไลน์อนุมัติทันทีที่ใช้เอกสารน้อยก็สามารถขอสินเชื่อออนไลน์ เมื่อทำการยื่นเอกสารในการสมัครครบถ้วนแล้วจะทราบผลไม่เกิน 7 วันซึ่งคุณก็สามารถตรวจสอบว่า การขอสินเชื่อออนไลน์อยู่ในขั้นตอนไหนโดยสามารถตรวจสอบผ่านแอพกู้เงินออนไลน์นั้นได้เลย ทำให้คุณได้รับความสะดวกสบายในการกู้เงินด่วนออนไลน์ที่สามารถทำได้ทุกที่ทุกเวลา
แอพกู้เงินออนไลน์มีการจ่ายเงินก่อนสมัครกู้เงินด่วนออนไลน์ไหม?
ในการขอเงินกู้ออนไลน์ผ่านทางแอพจะมีให้กรอกข้อมูลส่วนตัวซึ่งจะมีขั้นตอนในการสมัครแอพกู้เงินออนไลน์อย่างชัดเจนแต่ถ้าแอพกู้เงินออนไลน์มีการเรียกเก็บค่าดำเนินการหรือที่เรียกว่า ค่าสมัครในการขอกู้เงินด่วนออนไลน์ในการสมัคร ขอให้คุณคิดไว้เลยว่าแอพกู้เงินออนไลน์อาจจะหลอกลวงเพื่อที่จะเอาข้อมูลของคุณไปใช้ในทางผิดกฎหมายซึ่งอาจจะนำความเสื่อมเสียมาให้แก่ตัวคุณ ดังนั้นควรจะมีการตรวจสอบให้ชัดเจนก่อนว่าแอพกู้เงินออนไลน์นั้นเป็นแอพที่ถูกกฎหมาย ถ้าแอพกู้เงินออนไลน์ที่ถูกกฎหมายก็สามารถสมัครกู้เงินออนไลน์ได้ทันที โดยที่คุณไม่ต้องกังวลว่า ข้อมูลส่วนตัวของคุณจะไปอยู่ในมือของมิจฉาชีพ มีหลายคนที่ได้หลงไปใช้บริการแอพสินเชื่อออนไลน์อนุมัติทันทีส่งผลให้คนนั้นต้องดำเนินคดีแจ้งความกับแอพผิดกฎหมายนั้นเพราะแอพสินเชื่อหมุนเวียนส่วนบุคคลนั้นมีการทวงหนี้โหดและมีการคิดอัตราดอกเบี้ยที่สูงเกินกว่ากฎหมายได้กำหนดไว้ โดยจะแตกต่างจากหน้าแอพที่มีการสมัครในครั้งแรกดังนั้นการตรวจสอบแอพกู้เงินออนไลน์ควรจะตรวจสอบก่อนการสมัครทุกครั้งหรืออาจจะหาข้อมูลแอพกู้เงินออนไลน์ผ่านเว็บไซต์รีวิวชื่อดังก็ได้เช่นกัน
เปิด 2 แหล่งของการกู้เงินออนไลน์ในปี 2567/2024
แหล่งกู้เงินด่วนออนไลน์กับสินเชื่อออนไลน์อนุมัติทันทีที่กำลังเป็นที่น่าสนใจคงจะไม่พ้นสินเชื่อกรุงไทยใจป้ำที่สามารถขอกู้เงินออนไลน์ได้ทุกอาชีพเพียงแค่มีเงินเดือน 15,000 บาทขึ้นไป โดยวงเงินในการสินเชื่อออนไลน์อนุมัติทันทีอย่างกรุงไทยใจป้ำจะให้วงเงินสูงสุด 5 เท่าของรายได้เฉลี่ยต่อเดือน การขอสินเชื่อออนไลน์จะไม่ใช้หลักทรัพย์หรือบุคคลในการค้ำประกัน วิธีกู้เงินกรุงไทยใจป้ำสามารถทำการกู้เงินออนไลน์ผ่านแอพ Krungthai NEXT ขั้นตอนในการสมัครสินเชื่อกรุงไทยใจป้ำสามารถสมัครได้ง่ายมากใช้เวลาไม่นานก็ทราบผลในการอนุมัติวงเงินแล้ว วิธีคิดดอกเบี้ยเงินกู้ของสินเชื่อกรุงไทยใจป้ำกรณีที่คุณเป็นผู้ประกอบการรายย่อยจะมีการคิดดอกเบี้ยร้อยละ 22 ต่อปีแต่ถ้าเป็นพนักงานประจำจะมีการคิดอัตราดอกเบี้ยร้อยละ 20 ต่อปีเท่านั้นซึ่งผู้ที่เป็นพนักงานประจำจะมีการคิดดอกเบี้ยต่ำมากกว่าผู้ประกอบการรายย่อย ส่วนระยะเวลาการในผ่อนชำระกู้เงินออนไลน์ได้จริงสามารถเลือกได้ตั้งแต่ 12-60 งวดซึ่งคุณก็สามารถเลือกงวดที่ต้องการชำระเงินได้แต่สินเชื่อกรุงไทยใจป้ำจะมีการผ่อนชำระเงินในทุกเดือน หากคุณสนใจที่จะสมัครกู้เงินออนไลน์ให้ทำการดาวน์โหลดแอพ Krungthai NEXT ผ่านระบบแอนดรอยด์และระบบ ios ได้เลย
ช่องทางในการติดต่อแอพกู้เงินออนไลน์ 2567/2024
หากคุณต้องการจะติดต่อเพื่อสอบถามเกี่ยวกับการขอกู้เงินออนไลน์สามารถติดต่อสอบถามได้ที่หมายเลขโทรศัพท์ที่ขึ้นไว้ในแอพกู้เงินออนไลน์หรือมีข้อสงสัยก็สามารถสอบถามเจ้าหน้าที่ได้ โดยวิธีกู้เงินแต่ละแอพกู้เงินออนไลน์อาจจะทำให้คุณเกิดข้อสงสัยก็สามารถสอบถามเราได้เลย คุณอาจจะกรอกข้อมูลที่ต้องการจะสอบถามหรือมีข้อสงสัยเกี่ยวกับแอพเงินกู้ได้จริง-บริการกู้เงินด่วนออนไลน์เพื่อให้เจ้าหน้าที่ติดต่อกลับก็ได้เช่นกัน
บทความให้ความรู้
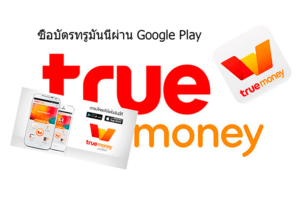
ขั้นตอนการซื้อบัตรทรูมันนี่ออนไลน์ผ่านช่องทางต่างๆ ทั้งเว็บไซต์หรือแอพ
ซื้อบัตรทรูมันนี่ผ่าน Google Play มีประโยชน์อย่างไร
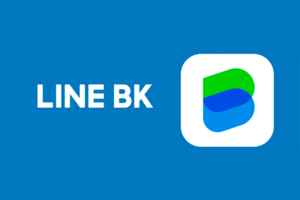
วิธีสมัคร line bk กสิกร พร้อมแนะนำวิธีสมัครให้ผ่านง่ายๆ ไม่กี่ขั้นตอน
สมัคร line bk มีข้อดีอย่างไร
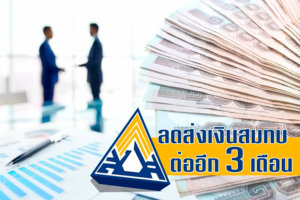
คำนวณเงินสมทบประกันสังคม 2567 ผ่านกองทุนประกันสังคมเพื่อดูว่าคุ้มไหม?
ทำความรู้จักกับเงินสมทบประกันสังคมคืออะไร คุ้มไหมกับที่จ่ายไป
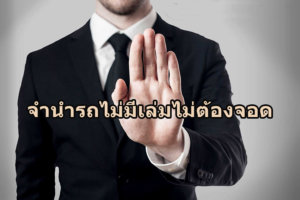
รับจํานํารถไม่มีเล่มไม่ต้องจอดที่ไหนดี พร้อมดูข้อดีข้อเสียไปพร้อมๆ กัน
จํานํารถไม่มีเล่มไม่ต้องจอดคืออะไร ทำได้จริงไหม มีข้อดีและข้อเสียอย่างไร และมีแหล่งบริการที่ไหนบ้างเช็คเลย